The transition to scaleup and beyond is significantly accelerated by enhancing intuition and entrepreneurial vision, which have been the early stage drivers, with a substantial amount of strategy driven by research and data.
But while corporate metrics (ARR/EBITDA etc.) are tracked from the early days, product and technology metrics are often hard to capture and come to fruition much later.
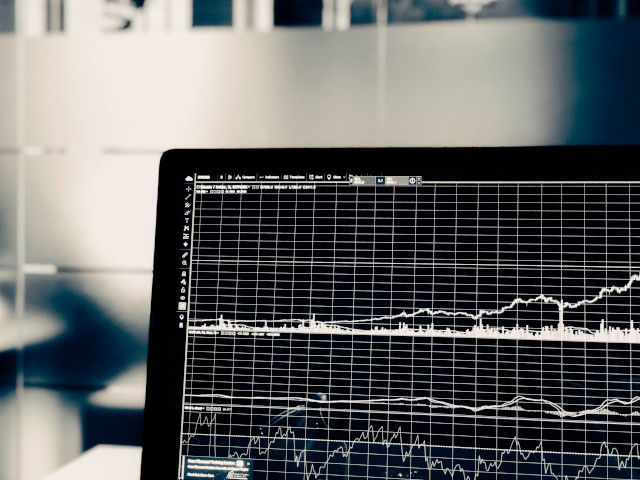
Why is it so hard?
Firstly, identifying the relevant product metrics is not straightforward all the time. While specific business models and products have a clear set of metrics from day one, others need few iterations to have enough clarity. Moreover, data quality and consistency across multiple data sets is not a trivial task and require bandwidth and a deliberate investment. If there’s no clarity on what to measure, such investment is a luxury not worth making.
Identifying Product Market Fit (PMF) is the demarcation line for accelerating your investment in analytics. Before PMF, data could be highly volatile, making the exercise extremely hard and prone to rework. At that stage, pragmatically instrument enough analytics at the feature level to assess if your experiments and hypothesis and look for correlation.
Once PMF is found, there should be clarity about what matters to users and to the business. It’s time to invest in your data analytics capabilities.
Don’t drink the cool-aid and buy into the idea that a single off the shelf solution will solve all your problems with “no developer time”. The creation of a data analytics capability is, first and foremost, an engineering heavy lifting exercise to have the correct data in the right place and at the right time. An off the shelf solution can give you building blocks, but those blocks still need to be assembled in line with your goals and data policies. That’s either via the vendor’s professional services or your internal teams.
Your newly born analytics capabilities will need 3 ingredients:
- A suitable infrastructure
- A flexible data exploration toolset
- An organisation design that will maximise your analytics initiative
Infrastructure for data analytics
The infrastructure for ETL/ELT will require architectural thinking. If you want analytics to be a differentiator, don’t treat it as a side project and invest at the beginning to have the proper foundation in place. A team will need to own the problem space, analyse current and potential future needs and all the cross-functional requirements (GDPR, security, etc.) to identify a solution with the necessary flexibility to grow with the rest of your business.
Tools for analysts
Once you have a data platform, think about how to enable different analytics approaches. As often with technology, optionality is the key. Analysts should have access to raw data for advanced analysis with R R or similar, while end-users will benefit from online dashboards and offline reports.
Organisational design for data-driven decisions
The last decision is around the organisational design around your newly defined capability. While the overall data platform’s governance might be owned by a team, the organic evolution should be distributed. Each product team should know how to add new data sources and access data for analysis. Your initial data team should act as your internal champion and enable the other groups via evangelism and improving developer and analyst experience.
To encourage a broader data-driven mindset, reduce the friction to access analyses. A matrix structure with a mix of central analysts pool for ad-hoc deep dives and analysts embedded in teams where data analyses are critical will deliver a great return.
Start the journey too early, and you’re committing to rework and frustration. Start at the right time, and you’ll experience acceleration in your decision making.